Research
Research Funders
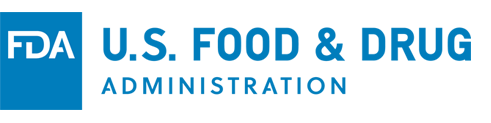
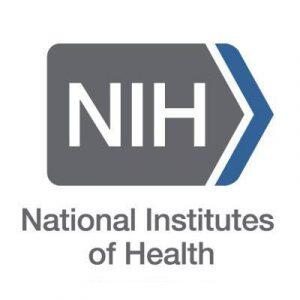
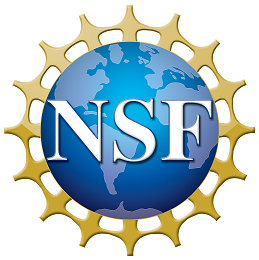
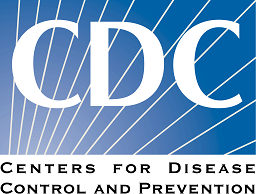

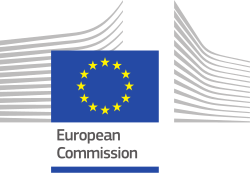
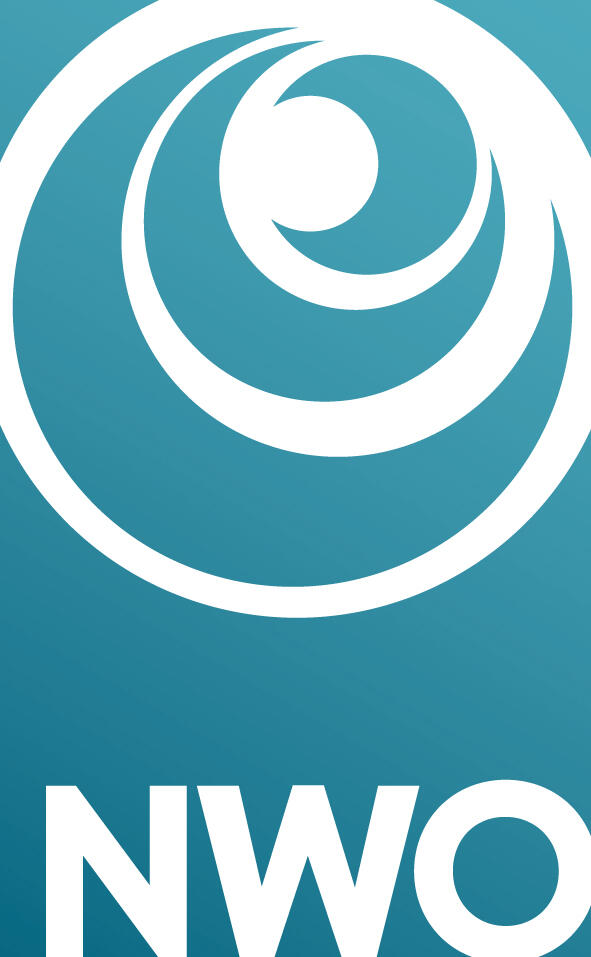

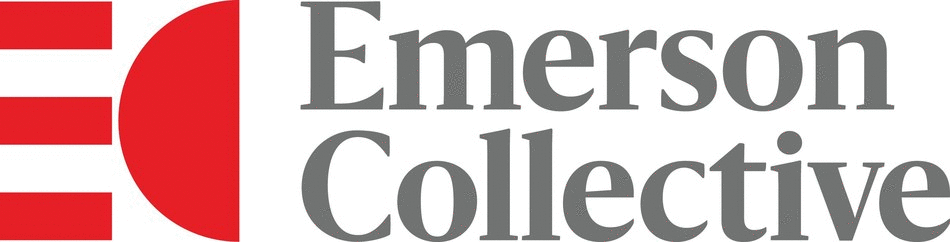
Affiliated with
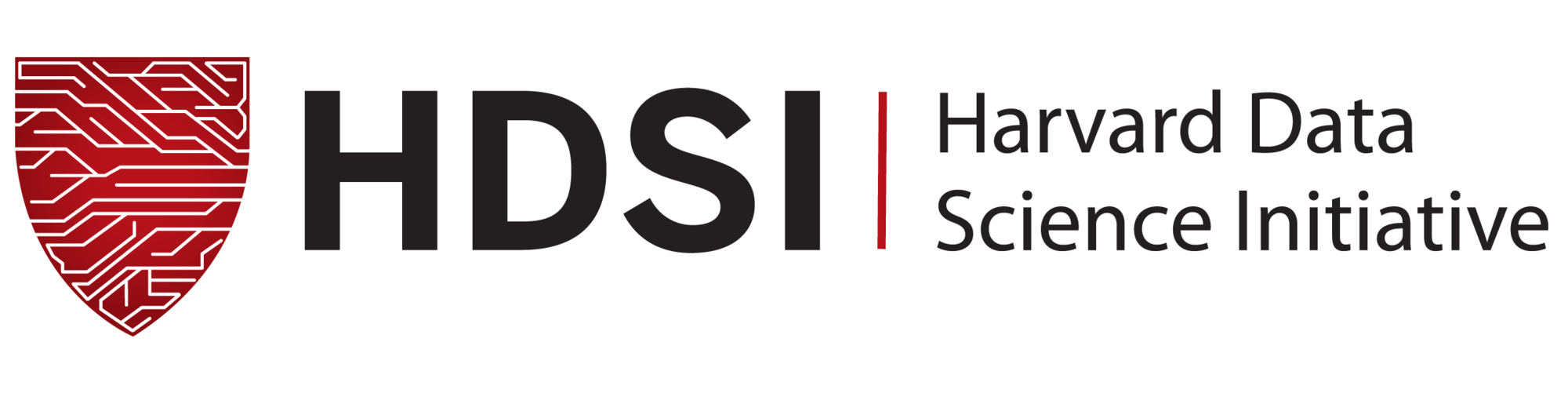
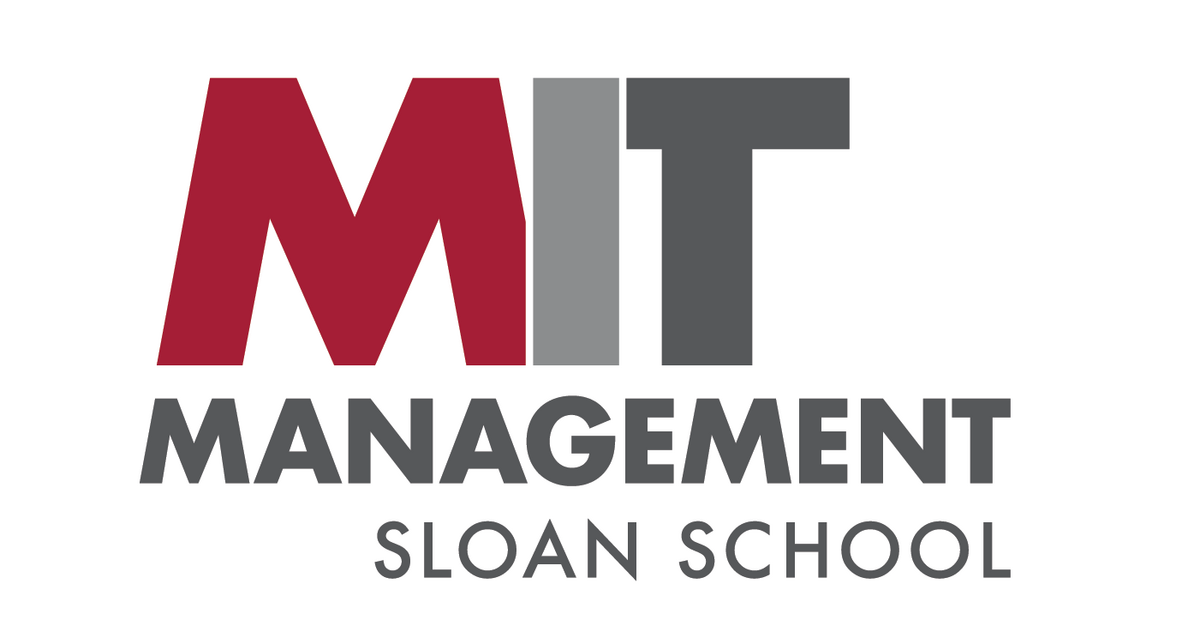
At MJ LAB, we are driven by three primary goals.
Firstly, our focus revolves around conducting simulation modeling and informatics research that pertains to various population-based health policies. In our modeling research, we draw upon optimization and strategy theories to assess the impacts of large-scale policies related to prevention, screening, and treatment. Notably, we have developed models for opioids, alcohol use, obesity, and post-traumatic stress disorder.
Secondly, we dedicate our research efforts to understanding the mechanisms that link human decision-making to healthcare systems. We recognize that numerous policy-resistant problems arise within this intersection. Our objective is to gain insights into why many health policies fail to yield enduring results or even generate outcomes contrary to their intended goals.
Lastly, we employ data science approaches to delve into the root causes of public health issues. We strive to develop rigorous methods that effectively connect models with quantitative data. To this end, we not only conduct phenomenological research but also contribute methodologically to bridge gaps across various domains of methodology and application.
Major Funded Projects
Using system dynamics to enhance the FDA’s opioids systems model and address the ongoing crisis
FDA: 1 U01 FD006868-01, 2019-2021
Dr. Jalali’s role: Principal Investigator ($999,532)
Goal: To enhance and expand FDA’s opioid systems model to augment the capabilities of policy and decision makers to curb the opioid crisis.
Expanding FDA’s opioids systems model to conduct economic evaluations and outcome analyses of national opioid policies
FDA: U01 FD007064-01, 2020-2022
Dr. Jalali’s role: Principal Investigator ($1,249,790)
Goal: To work collaboratively with FDA to expand its current opioids systems model to include a cost-effectiveness analysis of interventions and policies to reduce opioid-related illnesses, including opioid misuse, use disorder, and overdose.
Monitoring multidimensional aspects of QUAlity of Life after cancer ImmunoTherapy, an Open smart digital Platform for personalized prevention and patient management (QUALITOP)
European Commission: 875171, 2020-2023
Dr. Jalali’s role: Lead Scientist of the MGH site; there are 13 sites in this project ($502,071)
Goal: To collect real-world quality-of-life data after cancer immunotherapy treatment and conduct causal inference analyses, identifying the determinants of immunotherapy-related adverse events. This will be accomplished using artificial intelligence and simulation modeling approaches.
Community based system dynamics models of alcohol and substance exposed pregnancy in northern plains American Indian women
NIH: 1R01DA050696-01, 2020-2024
Dr. Jalali’s role: Co-Investigator (PI: Dr. Deutsch)
Goal: To develop and utilize community-based system dynamics models on the issues of alcohol/substance exposed pregnancy, inter-partner violence, and unplanned pregnancies among American Indian women.
Enhancing FDA’s opioids systems modeling efforts to more comprehensively address fentanyl, stimulants, polysubstance use, and associated outcomes
FDA: U01FD00745501, 2021-2022
Dr. Jalali’s role: Principal Investigator ($1,249,222)
Goal: To expand the scope of our opioid system modeling efforts at FDA by adding detail regarding stimulant use, fentanyls adulteration of stimulants, and fentanyls tablets, allowing us to improve estimates of quality of life and overdose and overdose fatality rates and increase confidence in the projected effects of intervention strategies on these outcomes.
Optimizing HIV care in less developed countries
NIH: R37 AI058736-MERIT, 2003-2023
Dr. Jalali’s role: Co-Investigator (PI: Dr. Freedberg)
Goal: To develop innovative modeling optimization methods to identify the combinations of testing, treatment, and prevention strategies that will have the greatest impact on specific populations in different countries.
Innovation across the spectrum of pediatric HIV care
NIH: R01HD079214, 2014-2024
Dr. Jalali’s role: Co-Investigator (PI: Dr. Ciaranello)
Goal: To help clinicians, program planners, and policymakers identify the optimal use of scarce resources to improve long-term health for children with HIV.
A community-based systems science approach to assess risk and protective factors and improve the efficacy and equity of intervention strategies for stimulant use, use disorder, and overdose
CDC: TBD (R01), 2021-2024
Dr. Jalali’s role: Principal Investigator ($1,085,719)
Goal: To build a model to test how different strategies could reduce use disorders and overdose in two states – Massachusetts and South Dakota – with a focus on differences by urban and rural setting as well as race and ethnicity, using what we learn about these states to inform national policy.